Antigoni Georgiadou
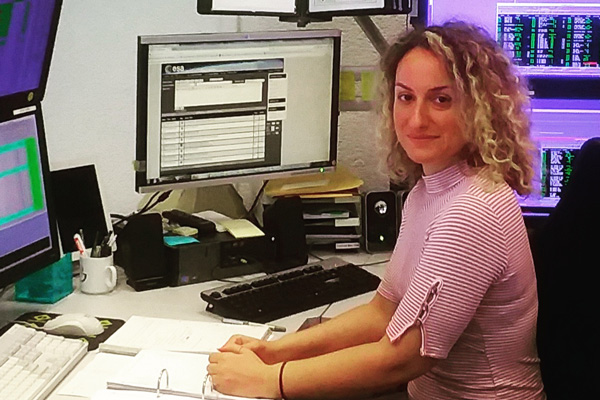
"Probe Universe as a Learning Machine."
College: Arts and Sciences
Degree Program: Applied and Computational Mathematics
Degree: Doctorate
Award: DAAD Short Term Research Grant (2017); URA Visiting Scholars Program (2017 & 2018)
Focus of research
My current research focuses on developing and applying versatile computational methods and optimization approaches for stellar evolution of core-collapse supernova progenitor models and cosmological simulations.
During the past three decades, cosmology turned from a highly theoretical discipline into a data-based, precision science. The increased size and quality of data from ongoing and future wide-field imaging surveys can play a pivotal role in uncovering the nature of dark matter and dark energy. At the same time, the exquisite precision of future surveys presents a serious challenge to theoretical modeling and interpretation of observational data. Until galaxy formation simulations become accurate, we will study the importance of baryonic physics on predictions of the matter power spectrum by mitigating (rather than modeling) the baryonic effects. A substantial bias in cosmological constraints from future surveys will not be accounted for without a mitigation technique.
Impact of Fellowships and Awards on Graduate Education
The Universities Research Association Visiting Scholars Program supported my work with the Theoretical Astrophysics group at the Fermi National Accelerator Laboratory in Batavia, Illinois. I was funded to apply a general framework to incorporate systematic effects into a likelihood analysis. My work at Fermilab started by performing a set of numerical simulations that sample a multi-dimensional parameter space of baryonic effects. For the analysis, we used the simulation set as a training sample and applied PCA parametrization and neural networks methods to model cosmological simulation parameters to develop an efficient interpolation technique that allows us to accurately predict, and subsequently mitigate, baryonic effects anywhere in the simulation parameter space.
It was important to work closely with my collaborators at Fermilab, as it boosted the efficiency and productivity of this analysis. It was a good match. I brought to the Theoretical Astrophysics Group at Fermilab my expertise with machine learning methods and emulators as a way to constrain parameters from limited simulation data. At the same time, my participation in this work played a major role in broadening my scientific expertise - an opportunity many graduate students do not have. Without the URA Visiting Scholarship support, the work would not have been a success. The impact of the URA Visiting Scholarship has greatly contributed to my progress as a graduate student, the Fermilab scientists, and the research project.